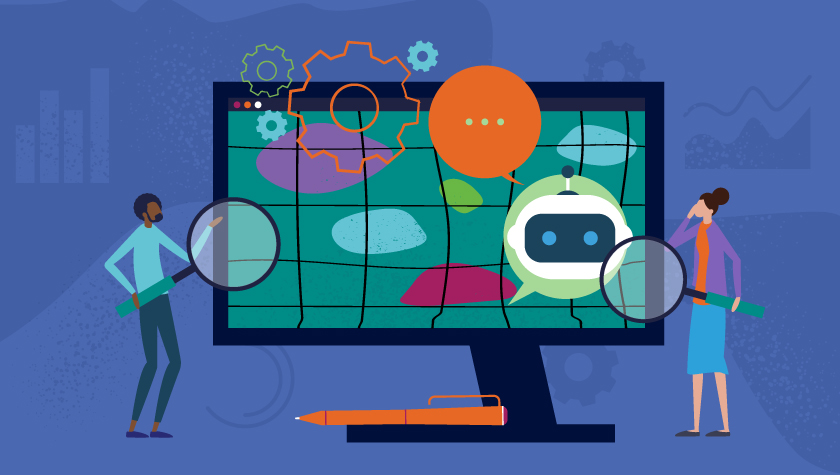
ChatGPT can spit out a fraudulent email from Amazon in a matter of seconds. It can pass an Ivy League business school’s MBA program. And it can even write a blog - like this one - with just a few clicks. These capabilities are going to make it much harder for everyday people to distinguish what is real from what is fake. This means ChatGPT is going to create a host of challenges and opportunities for the data science community.
Text generated by ChatGPT is in italics.
I may be a data scientist, but I’m also human. So of course, I asked ChatGPT to answer the questions I had about how it will impact the data science field.
Here’s what it had to say:
Question: What is the implication of Generative AI on data science?
“Generative AI, such as Generative Adversarial Networks (GANs) and Variational Autoencoders (VAEs), has the potential to impact data science in several ways. One of the most significant implications is the ability to generate synthetic data. This can be useful for a variety of tasks, such as training machine learning models, creating simulations, and augmenting limited datasets. Additionally, generative models can be used for tasks such as anomaly detection, image and text generation, and even drug discovery. However, it is important to note that the use of generative AI also raises ethical and societal concerns, such as the potential for misuse in areas such as deepfake generation.”
That last point is certainly worth unpacking further. ChatGPT is clearly capable of creating content rapidly. But a great deal of that content may not be true. And that’s where we need to be careful about how we use it – including in the data sciences.
ChatGPT-ing is the new ‘Googling’
Through a data science lens, one of the most concerning parts of Generative AI like ChatGPT is the lack of a second opinion. Ask it any question and not only does it answer you quickly, but it also writes high-quality responses with authority. After witnessing both the speed and authority of its responses, it’s challenging to push back against its answers.
No wonder Big Tech giants like Google are pushing the “code red” button. After all, the program is going through a similar evolution to Google’s. When the search engine first debuted, some people were slow to adopt it. They could just check the yellow pages or a book for information. But it only took a few years for Google to become such an invaluable source of information that it morphed into a verb – Googling. The term is now part of our everyday parlance. Need to learn something fast? Just Google it! “How did you know that? I Googled it!”
Google’s ease of use had an unfortunate side effect. It significantly lowered the entry barrier to becoming an expert in any field. As a result, one of Google’s biggest strengths – its ease of use – has become one of its biggest liabilities. It became easy for misinformation and fake news to spread online.
ChatGPT is going through a similar phase as Google. It’s trained on data that only goes to 2021, some of which may be outdated, include human biases, or even inaccurate information. Just as it proved risky to treat Google as an unchallenged source of truth, it’s important to treat ChatGPT the same way.
The 4 Key Data Science Challenges of ChatGPT
Given these capabilities, ChatGPT is poised to significantly impact both the data science field and broader society in three key ways.
1. It’s harder to distinguish what’s real
Creating text-based content isn’t the limit of Generative AI’s capabilities. We’ve already discussed how the technology can be used to generate phishing emails, deepfakes, audio, and images. The technology can manipulate or fabricate images, videos, and audio with ease. This makes it 100 times harder to spot fake news or misinformation when images can be repurposed or invented on the spot. Over time, the model could further propagate misinformation. This makes it harder to trust the data because you risk building a model that introduces bias into your model’s decision-making.
2. It will automate or replace certain data tasks
This point isn’t necessarily a negative. Let’s say you can’t make an informed prediction about a group or sample because you lack data. Generative AI can create synthetic data out of thin air to represent your target population. This is actually a common technique in data science and Generative AI could accelerate its practice.
However, data scientists will have to look at the generated data to ensure it is representative of real-life data. If the data generated under-represents certain groups, it could lead to unfair decisioning or inaccurate reports.
Generative AI could automate certain data-handling tasks, including cleaning large volumes of text data. It can also enhance anomaly detection making it easier to spot new fraud patterns. However, data scientists must constantly monitor these models to ensure they work as intended.
3. No profession is safe
Generative algorithms have almost everyone in every profession on edge. And with good reason. The technology could very well disrupt every profession from content creators like digital artists, bloggers, and journalists to knowledge-based professions like doctors, architects, engineers, and even lawyers. While this is a scary prospect, it’s also an exciting one. This technology can democratize so many things at once. It can write a story you have in your head in a few seconds, compose a song, or create a design with just a few descriptive words.
4. It creates a single source of truth
We’ve already seen technology unintentionally craft “echo chambers” by giving users information that aligns with their views. ChatGPT could accelerate that trend. Some people will disregard dissenting views or forfeit facts in favor of the outputs generated by ChatGPT that align with their biases.
4 Generative AI Guardrails for Data Scientists
Like all other professions, Generative AI will have a big impact on the data science field as well. That’s why data scientists should adopt guardrails to ensure the integrity of their work. As the technology becomes more prevalent, data scientists should adopt the following framework:
- Make sure data is reliable, valid, and bias-free: As we’ve noted, Generative AI is capable of creating data out of thin air. But it lacks the capacity to understand if what it has created is accurate or true. Data scientists must review the synthetic data and compare it with real-life data to confirm if it’s trustworthy. For example, always check the underlying data distributions and see if bias is introduced in the data or by evaluating the performance of a model trained on the generated data.
- Embrace Explainability and Watch for AI Bias: Generative AI needs to be able to explain itself at each step. As we mentioned, the system doesn’t understand if it includes biased data. That’s why it needs to be able to explain its decisions in a way that makes it more transparent. Data scientists need to teach AI how to detect biases and make sure the data it uses is representative of the real world.
- Implement security guidelines: As data scientists, we have an obligation to make sure anything created by Generative AI is not used for malicious purposes. Look at what happened with deepfake technology. Scientists created it to generate realistic video duplicates of real people. But now the technology is easily available and used in fraud. You may not intend for your creation to be used maliciously, but without the proper security guardrails in place, that’s what could happen.
- Think ethically: As the adoption of this technology continues, we have to stay focused on our ethical obligations. Generative AI is already raising questions about intellectual property and plagiarism and demonstrating how it can be abused for fraud. As data scientists, we must always remember our ethical obligations.
Even as a data scientist, I wondered if ChatGPT is coming for my job. So I asked it:
“Generative AI has the potential to augment the work of data scientists, but it is unlikely to completely replace them in the near future. While AI models can be trained to perform various tasks, such as data analysis and prediction, they still require human oversight and interpretation.”
Phew! For now, at least…
Share this article:
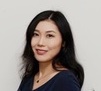
Xin Ren
Xin Ren is the Head of Risk & AI for NAMER and APAC at Feedzai. She has been working in the Financial industry for over 10 years and specialized in delivering AI-based strategy and bringing data science best practices to the clients.
Related Posts
0 Comments15 Minutes
ChatGPT Revolutionizes Retail Banking…Soon
The world is buzzing with excitement about Large Language Models (LLM) and Generative AI…
0 Comments8 Minutes
Understanding FairGBM: Feedzai’s Experts Discuss the Breakthrough
Feedzai recently announced that we are making our groundbreaking FairGBM algorithm…
0 Comments5 Minutes
Feedzai Releases and Open Sources FairGBM Algorithm
Today, we’re thrilled to announce the next innovation in our commitment to delivering…